Mapping relationships between Neo4j spatial nodes with GeoPandas
Previously, we mapped neo4j spatial nodes. This time, we want to take it one step further and map relationships.
A prime example, are the relationships between GTFS StopTime and Trip nodes. For example, this is the Cypher query to get all StopTime nodes of Trip 17:
MATCH
(t:Trip {id: "17"})
<-[:BELONGS_TO]-
(st:StopTime)
RETURN st

To get the stop locations, we also need to get the stop nodes:
MATCH
(t:Trip {id: "17"})
<-[:BELONGS_TO]-
(st:StopTime)
-[:STOPS_AT]->
(s:Stop)
RETURN st ,s
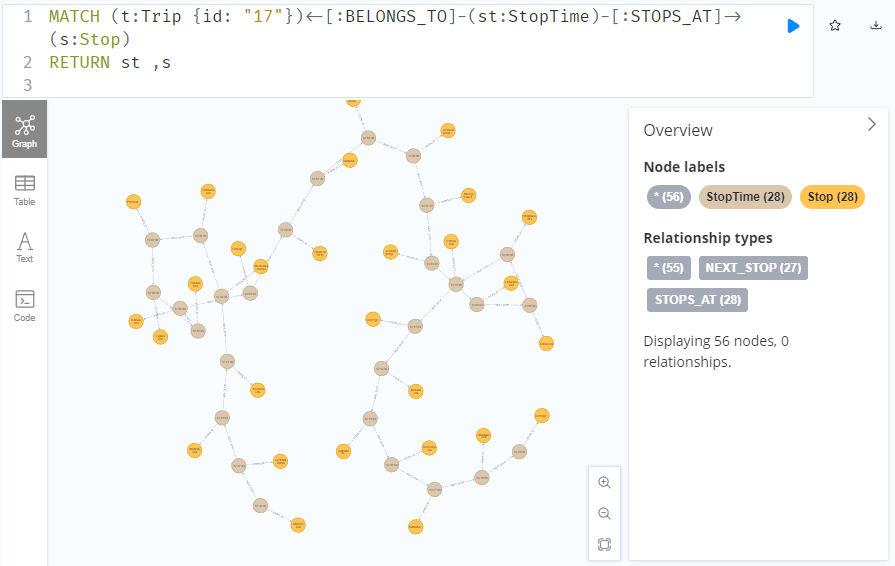
Adapting our code from the previous post, we can plot the stops:
from shapely.geometry import Point
QUERY = """MATCH (
t:Trip {id: "17"})
<-[:BELONGS_TO]-
(st:StopTime)
-[:STOPS_AT]->
(s:Stop)
RETURN st ,s
ORDER BY st.stopSequence
"""
with driver.session(database="neo4j") as session:
tx = session.begin_transaction()
results = tx.run(QUERY)
df = results.to_df(expand=True)
gdf = gpd.GeoDataFrame(
df[['s().prop.name']], crs=4326,
geometry=df["s().prop.location"].apply(Point)
)
tx.close()
m = gdf.explore()
m
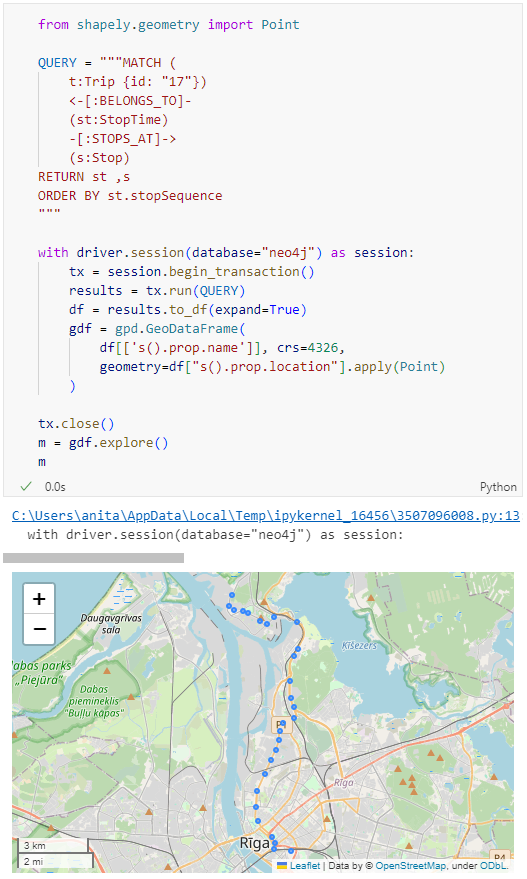
Ordering by stop sequence is actually completely optional. Technically, we could use the sorted GeoDataFrame, and aggregate all the points into a linestring to plot the route. But I want to try something different: we’ll use the NEXT_STOP relationships to get a DataFrame of the start and end stops for each segment:
QUERY = """
MATCH (t:Trip {id: "17"})
<-[:BELONGS_TO]-
(st1:StopTime)
-[:NEXT_STOP]->
(st2:StopTime)
MATCH (st1)-[:STOPS_AT]->(s1:Stop)
MATCH (st2)-[:STOPS_AT]->(s2:Stop)
RETURN st1, st2, s1, s2
"""
from shapely.geometry import Point, LineString
def make_line(row):
s1 = Point(row["s1().prop.location"])
s2 = Point(row["s2().prop.location"])
return LineString([s1,s2])
with driver.session(database="neo4j") as session:
tx = session.begin_transaction()
results = tx.run(QUERY)
df = results.to_df(expand=True)
gdf = gpd.GeoDataFrame(
df[['s1().prop.name']], crs=4326,
geometry=df.apply(make_line, axis=1)
)
tx.close()
gdf.explore(m=m)

Finally, we can also use Cypher to calculate the travel time between two stops:
MATCH (t:Trip {id: "17"})
<-[:BELONGS_TO]-
(st1:StopTime)
-[:NEXT_STOP]->
(st2:StopTime)
MATCH (st1)-[:STOPS_AT]->(s1:Stop)
MATCH (st2)-[:STOPS_AT]->(s2:Stop)
RETURN st1.departureTime AS time1,
st2.arrivalTime AS time2,
s1.location AS geom1,
s2.location AS geom2,
duration.inSeconds(
time(st1.departureTime),
time(st2.arrivalTime)
).seconds AS traveltime

As always, here’s the notebook: https://github.com/anitagraser/QGIS-resources/blob/master/qgis3/notebooks/neo4j.ipynb